Understanding the Importance of Data Labelling Services for Businesses
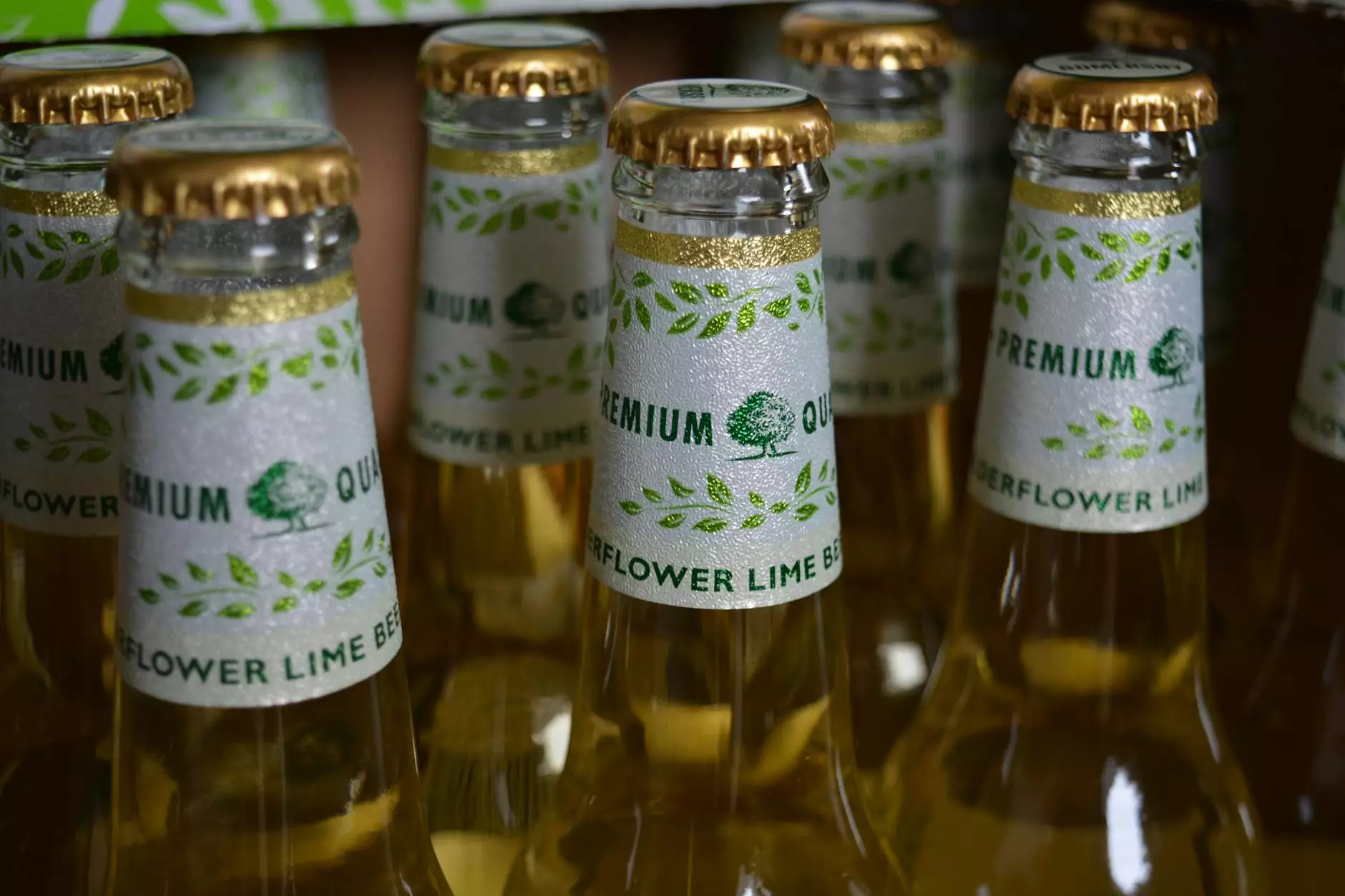
What is Data Labelling Service?
Data labelling service is a critical process in the field of machine learning and artificial intelligence (AI). It involves the meticulous organization and tagging of data, which allows AI algorithms to learn and make predictions more effectively. Essentially, data labelling transforms raw data into a structured format that machines can understand, thereby enhancing the outcomes of AI applications.
The Rising Demand for Data Labelling Services
With the rapid growth of AI technology, the demand for accurate and high-quality data labelling services has skyrocketed. Businesses across various industry sectors such as healthcare, automotive, finance, and retail are leveraging AI to improve their operations. Consequently, the need for robust and precise data labelling becomes paramount.
- Healthcare: Data labelling is used in medical image analysis, facilitating the diagnosis of diseases.
- Automotive: Self-driving car systems rely heavily on labelled data to discern objects and navigate safely.
- Finance: Algorithms are trained on labelled transaction data to detect fraudulent activities efficiently.
- Retail: Understanding customer behavior through labelled interaction data optimizes sales strategies.
The Data Labelling Process
The data labelling process can vary significantly depending on the nature of the data and the intended use. However, it generally follows these steps:
- Data Collection: Relevant data is gathered based on project requirements.
- Data Preprocessing: The collected data is cleaned and organized for labelling.
- Labelling: Human experts or automated systems tag the data based on predefined criteria.
- Quality Assurance: A review process ensures that the labelled data meets quality standards and is accurate.
- Feedback Loop: Continuous improvement is achieved through iterative feedback and retraining of the model.
Benefits of Using Data Labelling Services
Engaging a professional data labelling service offers numerous advantages that can significantly enhance a business's efficiency and output.
1. Improved Accuracy and Consistency
Utilizing a dedicated service ensures higher accuracy and consistency in data labelling. Human experts can apply nuanced understanding and context that automated systems may miss, resulting in better model performance.
2. Cost-Effectiveness
By outsourcing data labelling tasks, businesses can save on operational costs associated with hiring in-house staff. This allows resources to be directed towards core business functions while experts handle the complexities of data labelling.
3. Save Time
Data labelling is a time-consuming task. Partnering with a specialized service provider expedites the process, allowing quicker turnaround times for projects and faster deployment of AI models in business operations.
4. Access to Expertise
Data labelling services often come equipped with teams of specialists who possess industry-specific knowledge and experience, ensuring that the data is labelled correctly according to the latest trends and best practices.
The Role of Technology in Data Labelling
Modern data labelling services are increasingly utilizing technology to enhance their offerings. Technologies such as automation, machine learning, and artificial intelligence are being integrated into the data labelling process.
- Automation: Automates repetitive tasks, allowing human experts to focus on complex labelling requirements.
- Machine Learning: Algorithms can assist in pre-labelling data, which is then fine-tuned by human annotators.
- Artificial Intelligence: Helps analyze large volumes of data to identify patterns that assist in better labelling efficiencies.
Choosing the Right Data Labelling Service Provider
Selecting a capable data labelling service provider is crucial for the success of your AI initiatives. Here are some factors to consider:
1. Quality of Service
Research the provider's track record with previous clients. Ensure they maintain high standards in data quality and accuracy.
2. Scalability
Choose a service that can handle projects of various sizes and can scale according to your business needs.
3. Security and Confidentiality
Make sure that the data labelling provider has stringent security measures in place to protect your sensitive data.
4. Cost Structures
Understand their pricing model to ensure that it fits within your budget while still providing quality services.
5. Customer Support
A responsive customer support team can provide vital assistance during the labelling process, addressing issues as they arise.
Real-world Applications of Data Labelling Services
Data labelling services are being widely adopted by various industries. Here are some concrete examples:
1. Retail Industry Analytics
Retailers use data labelling to analyze consumer behavior and optimize inventory management systems. By labelling customer purchase data, they can segment customers based on preferences, leading to personalized marketing strategies.
2. Autonomous Vehicles
Companies in the automotive industry label vast amounts of video footage to train machine learning models for self-driving technology. This labelling process is critical for safely navigating complex environments.
3. Healthcare Diagnostics
Medical imaging technologies rely on accurate data labelling to identify patterns in x-rays, MRIs, and CT scans. Accurate data labelling in this field can dramatically improve diagnostic accuracy.
4. Financial Fraud Detection
Financial institutions label transaction data to develop algorithms that can detect fraudulent activities in real-time, safeguarding both consumers and businesses from potential losses.
The Future of Data Labelling Services
As AI continues to evolve, so too will the methodologies and technologies involved in data labelling services. Future trends include:
- Increased Automation: More processes will be automated, allowing for faster and more efficient data labelling.
- AI-Assisted Labelling: Improved algorithms will aid human annotators, reducing time while increasing accuracy.
- Collaborative Labelling Platforms: Systems that facilitate real-time collaboration between data scientists and labelers will emerge.
- Greater Focus on Quality Control: Enhanced techniques for ensuring labelled data quality will become standard practice to ensure that AI models are trained on the best data available.
Conclusion
In conclusion, the role of data labelling services in contemporary business is undeniable. As organizations increasingly rely on AI technologies to drive their operations, the significance of high-quality, well-structured data cannot be overstated. By investing in professional data labelling services, businesses can enhance their AI strategies, ultimately leading to significant improvements in operational efficiency and competitiveness in the market.
Exploring services provided by platforms like keymakr.com can provide valuable insights and assistance in navigating the complex landscape of data labelling in today's business world.